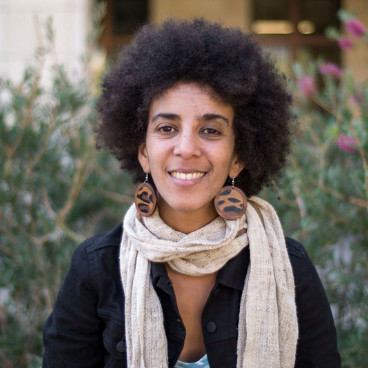
Timnit Gebru
Founder and Executive Director of DAIR
DAIR, Distributed AI Research Institute
Bio
Timnit Gebru is the founder and executive director of the Distributed Artificial Intelligence Research Institute (DAIR). Prior to that she was fired by Google in December 2020 for raising issues of discrimination in the workplace, where she was serving as co-lead of the Ethical AI research team. She received her PhD from Stanford University, and did a postdoc at Microsoft Research, New York City in the FATE (Fairness Accountability Transparency and Ethics in AI) group, where she studied algorithmic bias and the ethical implications underlying projects aiming to gain insights from data. Timnit also co-founded Black in AI, a nonprofit that works to increase the presence, inclusion, visibility and health of Black people in the field of AI, and is on the board of AddisCoder, a nonprofit dedicated to teaching algorithms and computer programming to Ethiopian highschool students, free of charge.Community rooted, independent AI research
The Distributed Artificial Intelligence Research Institute (DAIR) was launched in December 2021 by Timnit Gebru as a space for independent, community-rooted AI research, free from Big Tech’s pervasive influence. Gebru believes that the harms embedded in AI technology are preventable and that when its production and deployment include diverse perspectives and deliberate processes, it can be put to work for people, rather than against them. With DAIR, Gebru aims to create an environment that is independent from the structures and systems that incentivize profit over ethics and individual well-being. In this talk, Gebru will discuss why she founded DAIR and what she hopes this interdisciplinary, community-based, global network of AI researchers can accomplish. She will discuss the incentive structures that make it difficult to perform ethical AI research, and give examples of how DAIR is hoping to forge a different path.Online series: October 26th (Wednesday), 16:30 (UTC)
Website Twitter
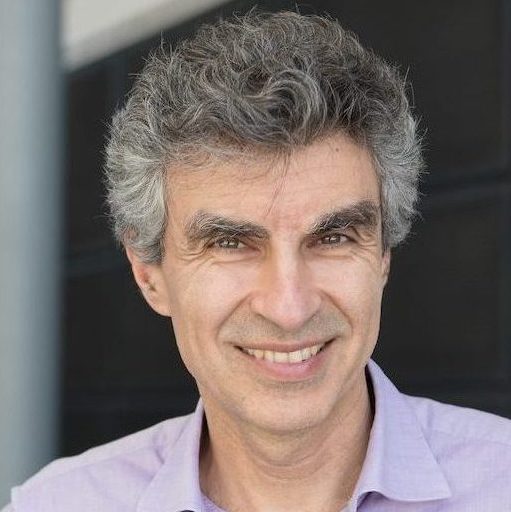
Yoshua Bengio
Scientific Director of Mila, Turing Award 2018
Mila, Quebec AI Institute · DIRO, Université de Montréal
Bio
Recognized worldwide as one of the leading experts in artificial intelligence, Yoshua Bengio is most known for his pioneering work in deep learning, earning him the 2018 A.M. Turing Award, “the Nobel Prize of Computing,” with Geoffrey Hinton and Yann LeCun. He is a Full Professor at Université de Montréal, and the Founder and Scientific Director of Mila – Quebec AI Institute. He co-directs the CIFAR Learning in Machines & Brains program as Senior Fellow and acts as Scientific Director of IVADO. In 2019, he was awarded the prestigious Killam Prize and in 2022, became the computer scientist with the highest h-index in the world. He is a Fellow of both the Royal Society of London and Canada, Knight of the Legion of Honor of France and Officer of the Order of Canada. Concerned about the social impact of AI and the objective that AI benefits all, he actively contributed to the Montreal Declaration for the Responsible Development of Artificial Intelligence.AI to explore molecular space and fight pandemics and climate change
Machine learning research is expanding its reach, beyond the traditional realm of the tech industry and into the activities of other scientists, opening the door to truly transformative advances in these disciplines. In this lecture I will focus on two aspects, modeling and experimental design, that are intertwined in the theory-experiment-analysis active learning loop that constitutes a core element of the scientific methodology. Computers will be necessary to go beyond the currently purely manual research loop and take advantage of high-throughput experimental setups and large-scale experimental datasets. I will discuss methods related to active learning, reinforcement learning, generative modeling, Bayesian ML, amortized variational learning and causal discovery. I will discuss the notion of epistemic uncertainty and how to estimate it. I will motivate generative policies that can sample a diverse set of candidate solutions to a problem, be it for proposing new experiments or causal hypotheses. Finally, I will describe current research to help us with these questions based on a new deep learning probabilistic framework called GFlowNets and how we plan to apply these in areas of great societal need like the unmet challenge of antimicrobial resistance or the discovery of new materials to help fight climate change.Online series: November 3rd (Thursday), 16:00 (UTC)
Website
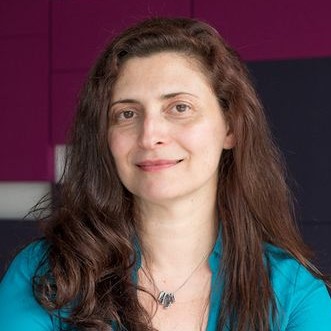
Irina Rish
Professor at Mila
Mila, Quebec AI Institute · DIRO, Université de Montréal
Bio
Irina Rish is a Full Professor in the Computer Science and Operations Research Department at the Université de Montréal (UdeM) and a core faculty member at MILA - Quebec AI Institute. She holds a Canada Excellence Research Chair (CERC) in Autonomous AI and a Canadian Institute for Advanced Research (CIFAR) Canada AI Chair. Before joining UdeM and MILA in 2019, Irina was a research scientist at the IBM T.J. Watson Research Center, where she worked on various projects at the intersection of neuroscience and AI. Dr. Rish received her MSc and PhD in AI from University of California, Irvine and MSc in Applied Mathematics from Moscow Gubkin Institute. Dr. Rish received multiple IBM awards, holds 64 patents, co-organized a great number of workshops, and served on several editorial boards and conference chairs. Over the past decades, her research has spanned multiple areas of AI, ranging from automated reasoning, neural data analysis to neuroscience-inspired AI. Currently, she is working on research areas such as continual learning, out-of-distribution generalization and robustness of neural networks, which she considers as essential for achieving the holy grail of the AI field, Artificial General Intelligence.Computational psychology and psychological computation: How AI and Brain Sciences can help each other
The rapidly developing field of computational psychology/psychiatry aims to bridge the gap between the “legacy” approaches still prevalent in mental health care that rely on primarily subjective self-reports and subjective evaluations by doctors - in a stark contrast to other scientific and medical fields where objective tests (e.g. blood work, X-ray, MRI) are typically used to provide an objective basis for diagnosis and prognosis. The promise of computational psychiatry is to bring psychological diagnosis to a more solid ground based on objective measurements, potentially including brain imaging, wearable sensor data, as well as statistical analysis of speech and text data - the are where modern AI techniques can prove highly useful, I will briefly mention some past and ongoing work by our group and collaborators in this directions, from developing more robust statistical models across brain imaging datasets, aiming at learning invariant features of a mental state or a person across varying setting, to transferring knowledge extracted across different data modalities, following recent advances in large-scale pretrained deep neural network models whose transfer capabilities appear to go far beyond what was previously considered possible. However, the road between AI and brain sciences is bidirectional: among many ways in which neuroscience, psychiatry and psychology can inspire novel AI approaches, the ability of an AI system to maintain “meaningful” dialog with a person, and reaching certain objectives of the dialog (e.g., application of AI in semi-automated therapy), can be considerably improved by importing certain practices and ideas from the therapeutic dialog into AI language models (I will briefly summarize our prior work on depression therapy dialogues). Finally, given the rapid advances in the capabilities of large scale models in the past 1-2 years, sometimes called a “scaling revolution” these days, also increase the importance of developing AI systems that are aligned with human values and can avoid harming humans while remaining useful to them - the blossoming field of AI Alignment (as a subfield of AI Safety, which, beyond alignment problem, includes robustness and interpretability of AI systems). The vast amount of practical knowledge from the fields of psychology and neuroscience, and from psychiatric therapy practice, can be clearly very advancing the AI Alignment field.Online series: November 7th (Monday), 16:00 (UTC)
Website Twitter
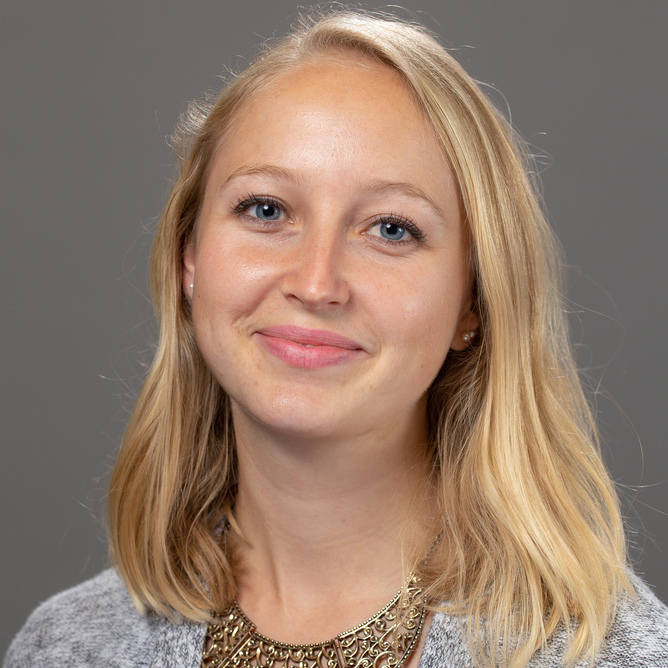
Hannah Kerner
Assistant Professor at the School of Computing and Augmented Intelligence at University of Maryland
School of Computing and Augmented Intelligence, University of Maryland · NASA, Harvest
Bio
Hannah Kerner is Assistant Professor in the School of Computing and Augmented Intelligence at the University of Maryland. Her research focuses on developing and advancing machine learning systems needed to address the world’s most pressing challenges, including food security, climate change and space exploration. As the machine learning lead and U.S. domestic co-lead for the NASA Harvest program, she is deploying research methods in real operations for stakeholders in industry, government and humanitarian organizations. In 2021, she was recognized on the Forbes 30 Under 30 list in science. Hannah Kerner also communicates challenges for developing ML applications for real-world problems and is passionate about advancing opportunities for people who have traditionally been underrepresented in computer science.AI and Earth observations for global agricultural monitoring and food security
Earth-observing satellites are collecting terabytes of observations of the entire planet every day with unprecedented clarity. These globally-available datasets offer immense opportunities for providing time-sensitive information needed by decision-makers about issues important to society, such as agricultural production and food security. Analyzing these petabyte-scale Earth observation datasets requires AI and machine learning methods designed to meet the unique challenges and opportunities of Earth observation data. Harvest is NASA’s applied sciences program on agriculture and food security, committed to advancing the use of satellite Earth observations to benefit food security and agriculture in the US and worldwide. In this talk, Harvest Director Inbal Becker-Reshef and Harvest ML/AI Lead Hannah Kerner will talk about how Harvest is using AI and Earth observations to rapidly respond to critical events impacting global agriculture and food security, including the Russian invasion of Ukraine.Online series: November 11th (Friday), 16:00 (UTC)
Website Twitter
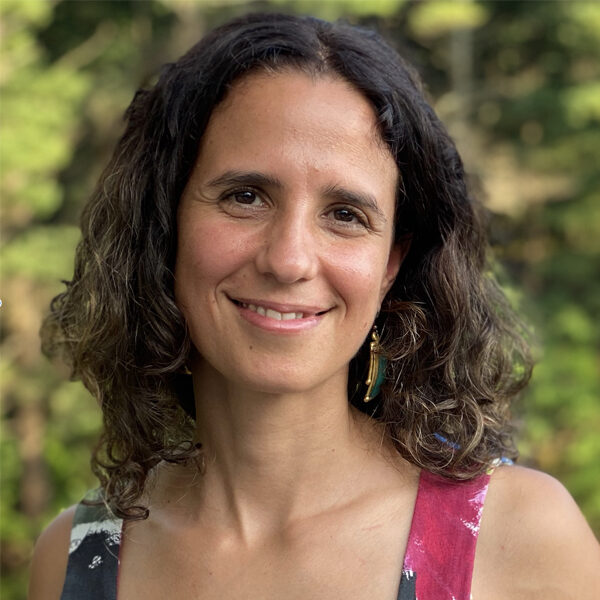
Inbal Becker-Reshef
Director of NASA Harvest, Professor at University of Maryland
NASA Harvest · Department of Geographical Sciences, University of Maryland
Bio
Dr. Becker-Reshef is the Director of NASA Harvest (NASA’s Applied Science Program on Food Security and Agriculture), and Research Professor at the University of Maryland. Her work is focused on the application of Earth Observations for agricultural monitoring from the field to global scales, supporting decisions in food security, sustainability, and agricultural markets. She worked closely with national and international partners to initiate the GEOGLAM (GEO Global Agricultural Monitoring) Program, adopted by the G20 in 2011 under the action plan on food price volatility and agriculture. Within this program she is a Program Scientists at the GEOGLAM Secretariat, leading the Crop Monitor initiative. She is a member of the G20 AMIS Steering Committee, UN 50X2030 Technical Advisory Group, US National Committee for IIASA, GEOGLAM-Committee on Earth Observation Satellites (CEOS) Technical Team, NASA Water Resources Applied Science Team, and led the NASA SERVIR Food Security and Agriculture Theme of the Applied Sciences Team (2016-2019). Her background is in soil sciences and remote sensing and she received her Ph.D in 2012 in Geographical Sciences from the University of Maryland. She was recognized by the US State Department, for her work on Food Security and Technologies winning US Asia-Pacific Economic Cooperation (APEC) Science Prize for Innovation, Research, and Education (ASPIRE) awarded by John Holdren, Former Assistant to the President for Science and Technology.AI and Earth observations for global agricultural monitoring and food security
Earth-observing satellites are collecting terabytes of observations of the entire planet every day with unprecedented clarity. These globally-available datasets offer immense opportunities for providing time-sensitive information needed by decision-makers about issues important to society, such as agricultural production and food security. Analyzing these petabyte-scale Earth observation datasets requires AI and machine learning methods designed to meet the unique challenges and opportunities of Earth observation data. Harvest is NASA’s applied sciences program on agriculture and food security, committed to advancing the use of satellite Earth observations to benefit food security and agriculture in the US and worldwide. In this talk, Harvest Director Inbal Becker-Reshef and Harvest ML/AI Lead Hannah Kerner will talk about how Harvest is using AI and Earth observations to rapidly respond to critical events impacting global agriculture and food security, including the Russo-Ukrainian war.Online series: November 11th (Friday), 16:00 (UTC)
Website Twitter
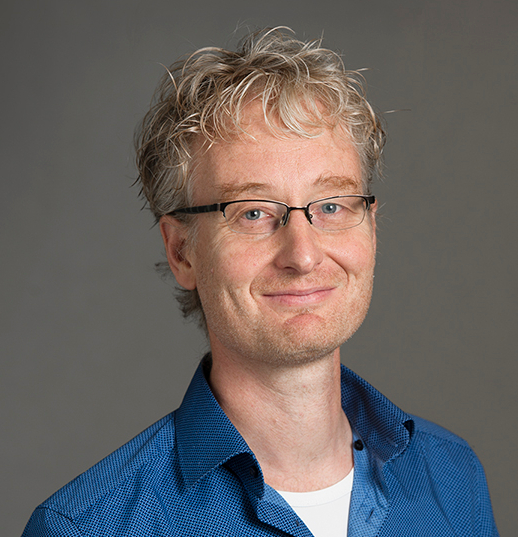
Max Welling
Director of AMLab, Co-Founder of Scyfer BV, Lead Scientist of the Microsoft Research Lab Amsterdam
AMLAB, University of Amsterdam · European Lab for Learning and Intelligent Systems (ELLIS)
Bio
Max Welling advanced the field of machine learning in multiple ways: Together with others he introduced variational autoencoders, graph neural networks and equivariant neural networks. He directs the Amsterdam Machine Learning Lab (AMLab) and co-directs the Qualcomm-UvA deep learning lab (QUVA) and the Bosch-UvA Deep Learning lab (DELTA). He also co-founded Scyfer BV and the lead scientist of the Microsoft Research Lab in Amsterdam. He is a fellow at CIFAR and ELLIS and a member of the advisory board of Neurips. Notably, Max Welling received the ECCV Koenderink Prize in 2010 and the ICML Test of Time award in 2021. He published well over 250 articles. His research aims to develop new machine learning methods in the areas of deep learning, Bayesian modeling and causal inference and apply those methods to large scale data domains in science and industry.Generating and steering molecules with ML and RL
After speech, text, image and video, a whole new application area is opening up for deep learning technology: accelerating molecular simulation. In this talk I will highlight two such methods in Transition Path Sampling and Ligand-Protein docking.Online series: November 14th (Monday), 16:00 (UTC)
Website Twitter
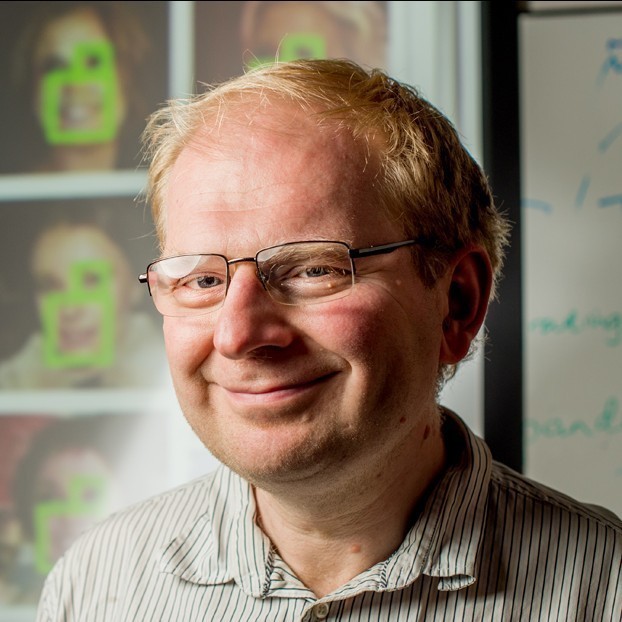
Alexei Efros
Professor at UC Berkeley
EECS Department, University of California, Berkeley · Berkeley Artificial Intelligence Research Lab (BAIR)
Bio
Alexei Efros is widely recognized for his ground-breaking research in computer vision and computer graphics. He is also very invested in self-supervised learning. Since 2013, Efros has been a professor at the EECS department at UC Berkeley. Before, he spend nine years at the Robotics Institute at CMU. His work was awarded with the ACM Prize in Computing in 2016. Among other awards, he received the Guggenheim fellowship (2008) and the Diane McEntyre Award for Excellence in Teaching Computer Science (2019). The goal of his research is to use vast amounts of unlabelled visual data to understand and model the visual world around us. He advocates for slow science. Efros openly supports the Ukraine since the beginning of the war.Visual self-supervision in the Post-Dataset Era
Starting in the early 2000s, datasets have revolutionized computer vision, arguably turning it from an art form into a science. But now, 20 years later, is our infatuation with fixed visual datasets and their training/test splits starting to cause harm? In this talk, I will describe some of our efforts to go past the fixed dataset paradigm and toward a more natural continuous online learning regime, where the distinction between training and test data is more blurred. I will present some of our latest work on test-time training, as well as a comically simple approach to test-time visual prompting / visual analogies.Online series: November 17th (Thursday), 19:00 (UTC)
Website
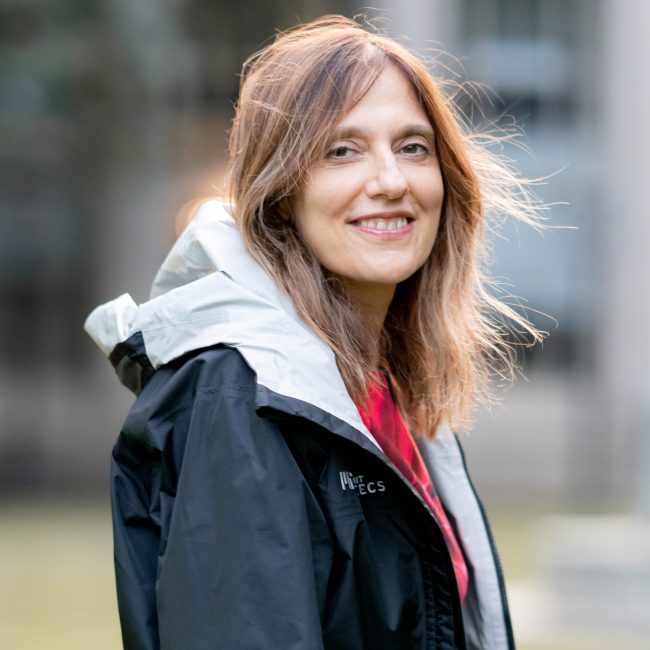
Regina Barzilay
Professor for AI and Health, Faculty Lead for AI at the MIT Jameel Clinic
School of Engineering, Computer Science and Artificial Intelligence Lab, Massachusetts Institute of Technology · Jameel Clinic, Massachusetts Institute of Technology
Bio
Regina Barzilay is famous for her break-throughs in deep learning for health. She was part of the team who discovered Halicin with deep learning, a new antibiotic compound that kills over 35 powerful bacteria, including AMR tuberculosis, and two of WHO's top-three most deadly bacteria. She is professor of electrical engineering and computer science at MIT and the faculty lead for AI at the MIT Jameel clinic, a research center advancing the field of AI health sciences. In 2017, Barzilay won the MacArthur Fellowship, known as the “Genius Grant”. Moreover, she became the first recipient of the $1 million AAAI Squirrel AI Award for Artificial Intelligence for the Benefit of Humanity (2020). After fighting breast cancer personally in 2014, Barzilay started applying machine learning to oncology, helping with early diagnosis, treatment and disease prevention.Expanding the reach of molecular models in the drug discovery space
Online series: November 21th (Monday), 16:00 (UTC)
Website Twitter
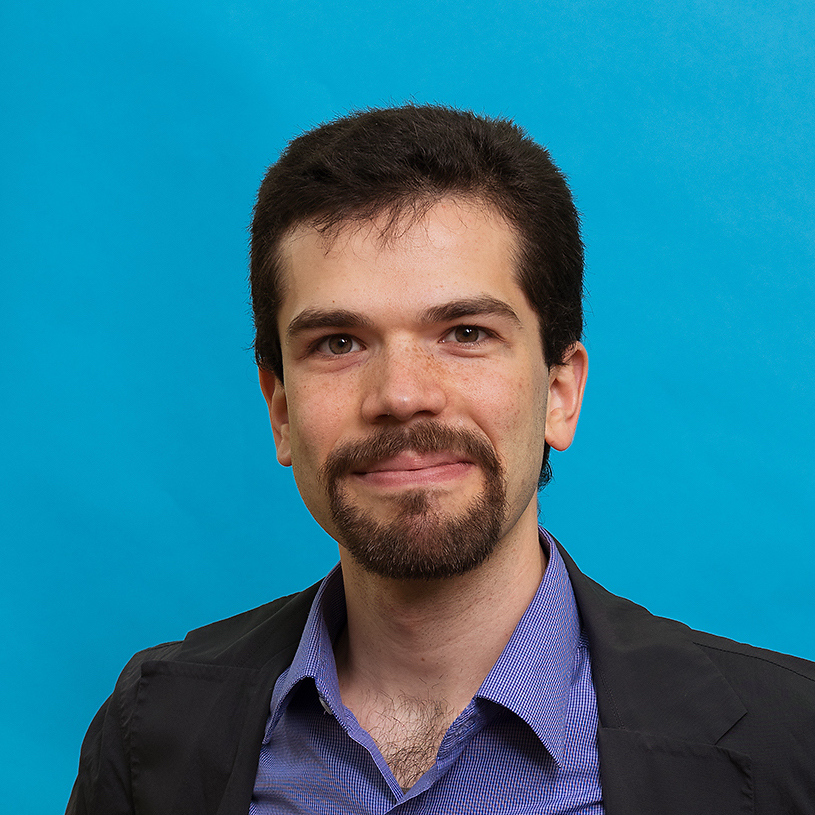
David Rolnick
Assistant Professor and Canada CIFAR AI Chair at McGill University
Mila, Quebec AI Institute · School of Computer Science, McGill University
Bio
David Rolnick is well known for his research on machine learning and climate change. This field was established by contributions such as his paper “Tackling Climate Change with Machine Learning”. Rolnick is an assistant professor at the School of Computer Science at the McGill University and holds a Canada CIFAR AI Chair. He is also co-founder and chair of Climate Change AI and a scientific co-director of Sustainability in the Digital Age. In 2021, he was named as one of the “35 Innovators Under 35” by the MIT Technology Review. He was a lead organizer of the first workshops on climate change at ICML, NeurIPS and ICLR, as well as th lead organizer of the first AI climate change event at COP25 (United Nations Climate Change Conference). David Rolnick develops new ML methods as tools for climate change adaptation and mitigation. Another part of his work is informing policy decision makers about the potential and limits of fighting climate change with AI.Machine learning in climate action
Machine learning (ML) can be a useful tool in helping society reduce greenhouse gas emissions and adapt to a changing climate. In this talk, we will explore opportunities and challenges in ML for climate action, from optimizing electrical grids to monitoring crop yield, with an emphasis on how to incorporate domain-specific knowledge into machine learning algorithms. We will also consider ways that ML is used in ways that contribute to climate change, and how to better align the use of ML overall with climate goals.Online series: November 24th (Thursday), 15:00 (UTC)
Website Twitter
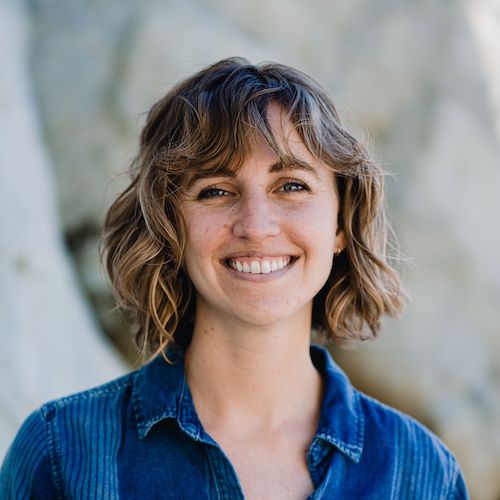
Sara Beery
Incoming Professor at MIT EECS's Faculty of AI and Decision Making and CSAIL
MIT EECS's Faculty of AI and Decision Making · Auto Arborist, Google
Bio
Sara Beery will join MIT as an assistant professor in the Faculty of Artificial Intelligence and Decision-Making in EECS in September 2023 and is currently a visiting researcher at Google working on urban tree mapping across North America. She received her PhD in computing and mathematical sciences at Caltech in 2022, where she was advised by Pietro Perona. Her research focuses on building computer vision methods that enable global-scale environmental and biodiversity monitoring across data modalities, tackling real-world challenges including strong spatiotemporal correlations, imperfect data quality, fine-grained categories, and long-tailed distributions. She partners with nongovernmental organizations and government agencies to deploy her methods in the wild worldwide and works toward increasing the diversity and accessibility of academic research in artificial intelligence through interdisciplinary capacity building and education.Mapping urban trees across North America with the Auto Arborist Dataset
Generalization to novel domains is a fundamental challenge for computer vision. Near-perfect accuracy on benchmarks is common, but these models do not work as expected when deployed outside of the training distribution. To build computer vision systems that solve real-world problems at global scale, we need benchmarks that fully capture real-world complexity, including geographic domain shift, long-tailed distributions, and data noise. We propose urban forest monitoring as an ideal testbed for studying and improving upon these computer vision challenges, while working towards filling a crucial environmental and societal need. Urban forests provide significant benefits to urban societies. However, planning and maintaining these forests is expensive. One particularly costly aspect of urban forest management is monitoring the existing trees in a city: e.g., tracking tree locations, species, and health. We introduce a new large-scale dataset that joins public tree censuses from 23 cities with a large collection of street level and aerial imagery, containing over 2.5M trees and 300 genera. This benchmark enables the exploration of automated urban forest monitoring across modalities and with respect to geographic distribution shifts, vital for such a system to be deployed at-scale.Online series: December 5th (Monday), 16:00 (UTC)
Website Twitter
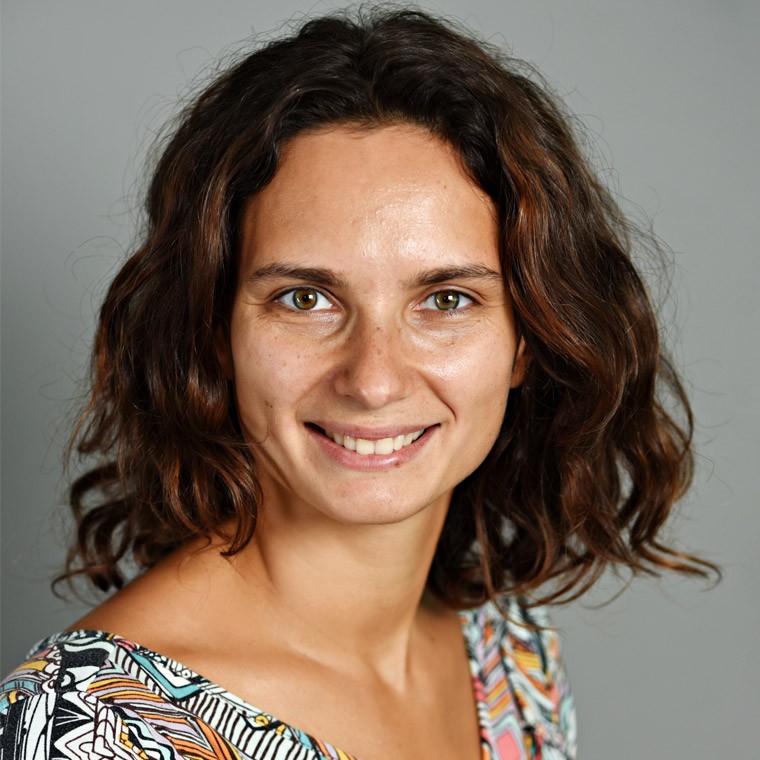
Anna Goldenberg
Professor at University of Toronto, Chair in Biomedical Informatics and Artificial Intelligence at the Hospital for Sick Children
Vector Institute for Artificial Intelligence · Department of Computer Science, University of Toronto · SickKids Research Institute
Bio
Anna Goldenberg is famous for her research on how machine learning can be used to map the heterogeneity seen in various human diseases. Her lab develops methodologies to identify patterns in collected data and improve patient outcomes. The “Similarity Network Fusion” she co-developed was the first patient data integration method that improved survival outcome predictions in different cancers. Goldenberg is professor in the departments of Computer Science and the Laboratory Medicine and Pathobiology at the University of Toronto. She is a Varma Family Chair in Biomedical Informatics and Artificial Intelligence at SickKids Research Institute as well as a CIFAR AI chair at the Vector Institute. Dr. Goldenberg trained in machine learning at Carnegie Mellon University, with a postdoctoral focus in computational biology and medicine. The current focus of her lab is on developing and deploying machine learning models to healthcare. Among other awards, Anna Goldenberg received the Early Researcher Award from the Ministry of Research and Innovation. Dr. Goldenberg’s lab is strongly committed to creating responsible AI to benefit patients across a variety of conditions.Time series ML for deployment in healthcare
There is growing support and excitement around AI integration into clinical practice. And yet, enabling AI in healthcare broadly is replete with many obstacles including fundamentally unresolved machine learning issues. In this talk I will cover several of our contributions to time series modeling including explainability, representation learning and generative modeling that we have worked on to date. I will also discuss some of the broader computer science contributions we had to make in order to get us closer to deployment. Finally, if time permits, I will touch on an important question of feedback loop, i.e. what happens when an ML solution is deployed and is starting to affect the labels that we are using to retrain and improve our models.Mila: Thursday, December 8th, 13:30 (EST)
Website
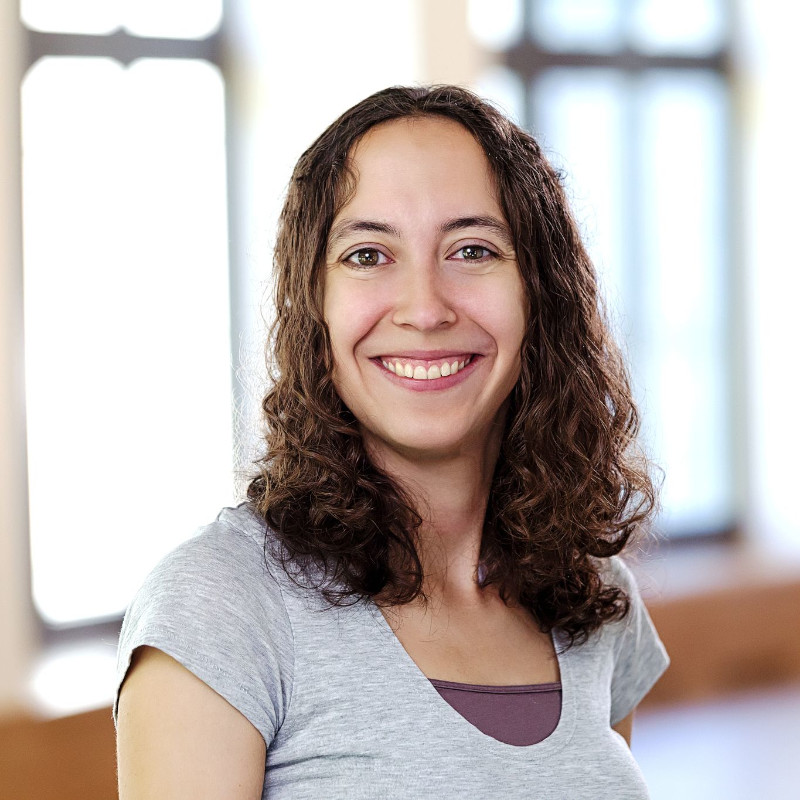
Olga Russakovsky
Assistant Professor at Princeton University
Department of Computer Science, Princeton University · Princeton Visual AI Lab
Bio
Dr. Olga Russakovsky is an Assistant Professor in the Computer Science Department at Princeton University. Her research is in computer vision, closely integrated with the fields of machine learning, human-computer interaction and fairness, accountability and transparency. She has been awarded the PAMI Young Researcher Award, the NSF CAREER award, the AnitaB.org's Emerging Leader Abie Award in honor of Denice Denton, the CRA-WP Anita Borg Early Career Award, the MIT Technology Review's 35-under-35 Innovator award, the PAMI Everingham Prize and the Foreign Policy Magazine's 100 Leading Global Thinkers award. In addition to her research, she co-founded and continues to serve on the Board of Directors of the AI4ALL nonprofit dedicated to increasing diversity and inclusion in Artificial Intelligence (AI). She was born in Kharkiv, Ukraine, and is "honored to be one of the keynote speakers -- while also being heartbroken that there's a need for the conference in the first place."Trustworthy (and trusted) computer vision
Computer vision is at an interesting cruxpoint: the systems are now both accurate enough that they're increasingly being deployed in high-stakes settings, but also have enough errors that folks are losing faith in them (with good reason). This talk will reflect on some of the recent work from the Princeton Visual AI lab on building more trustworthy (and more trusted) computer vision. I'll talk about improving the benchmarks, mitigating the social bias of the systems, and creating better model explanations that are more understandable for end users.Mila: Thursday, December 8th, 09:45 (EST)
Website
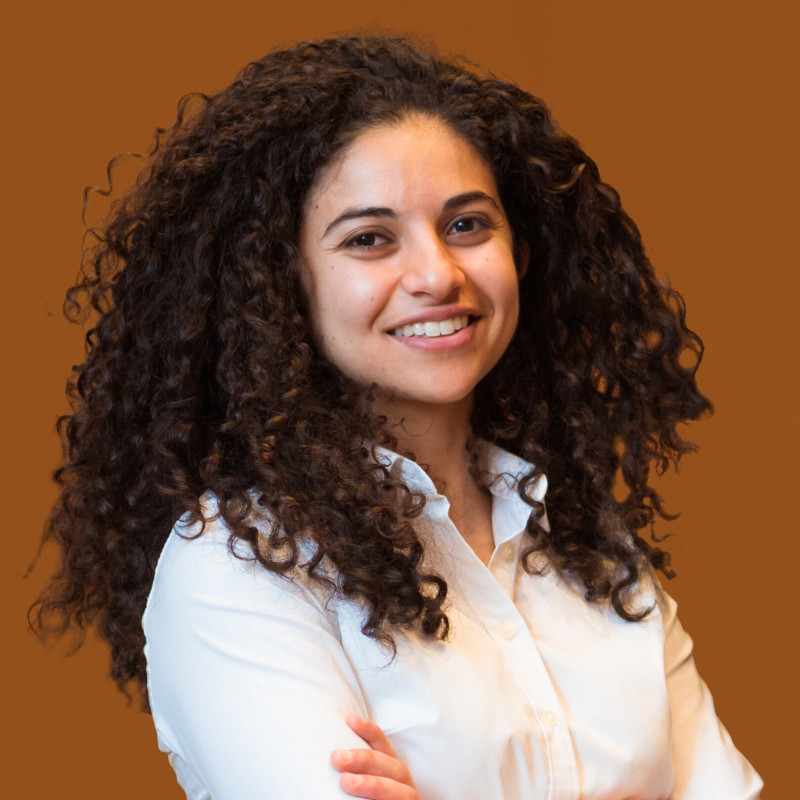
Ava Amini
Senior Researcher at Microsoft Research
Microsoft Research
Bio
Ava Amini is a Senior Researcher at Microsoft Research, New England. Her research focuses on developing new computational technologies for precision medicine, where she works at the interface of machine learning and biophysics. She completed her PhD in Biophysics at Harvard University, where she was supported by the NSF Graduate Research Fellowship, and her SB in Computer Science and Biology at MIT. Ava is passionate about machine learning outreach and leadership: she is a co-founder of MomentumAI, which provides all-expenses-paid programs for high schoolers to learn AI, and was a lead organizer and instructor for MIT Introduction to Deep Learning.AI to optimize biology
The potential of artificial intelligence (AI) in biology is immense, yet its success is contingent on interfacing effectively with wet-lab experimentation. In this talk, I will focus on two settings, biomolecular and experimental design, in which we’ve developed new AI algorithms to optimize biology and inform the experimental process. I will first share recent work in developing a diffusion-based generative model that designs protein structures by mirroring the native protein folding process. Moving from molecules to experiments, I will present a method — evidential deep learning — for uncertainty quantification in neural networks and demonstrate its potential to guide key steps in experimental lifecycles, opening the door for sustained feedback between computation and experimentation in the biological sciences.Mila: Thursday, December 8th, 15:00 (EST)
Website
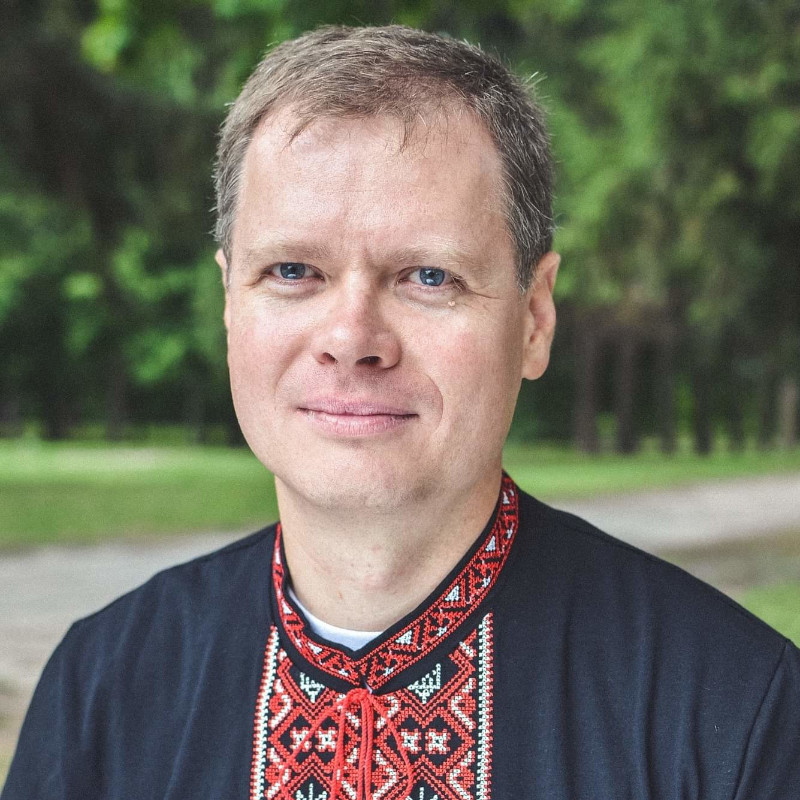
Oleksandr Romanko
Lead Research Scientist
SS&C Technologies Canada · University of Toronto · Ukrainian Catholic University · Kyiv School of Economics · American University Kyiv
Bio
Dr. Romanko is a Lead Research Scientist in Financial Risk Quantitative Research at SS&C Technologies Canada, he is also an Adjunct Professor at University of Toronto, Ukrainian Catholic University, Kyiv School of Economics and American University Kyiv. Dr. Romanko received Ph.D. in Computer Science from McMaster University. Previously, he worked as a Senior Research Analyst and Manager of Quantitative Research at Watson Financial Services division of IBM. Dr. Romanko received several industrial awards including IBM Outstanding Technical Achievement Award in 2014 and Eminence and Excellence IBM Award to Quantitative Research Group of IBM Risk Analytics in 2012. He also received a number of academic awards from INFORMS, Canadian Operational Research Society and Mitacs. Dr. Romanko is an author of more than 20 articles and book chapters in financial risk management, machine learning, analytics and operations research. His research focuses on optimization algorithms, financial portfolio optimization, machine learning and artificial intelligence applied to finance and risk management. To popularize data science and analytics as a new career option in Ukraine, starting from 2013 Dr. Romanko co-organized and taught more than ten one- and two-day courses in Data Science, Business Analytics and Artificial Intelligence with more than 5000 attendees. Dr. Romanko is a co-founder of Canada’s Mitacs Research Internship Program for Ukraine, that allowed more than 300 Ukrainian students to participate in research internship projects at Canadian universities. Dr. Romanko served as a Senior Policy Advisor to Anna Novosad, Minister of Education and Science of Ukraine in 2019-2020. He is also a member of the board of directors of Ukrainian Canadian Congress and Shevchenko Scientific Society of Canada.AI for Education
Mila: Thursday, December 8th, 16:00 (EST)
Website
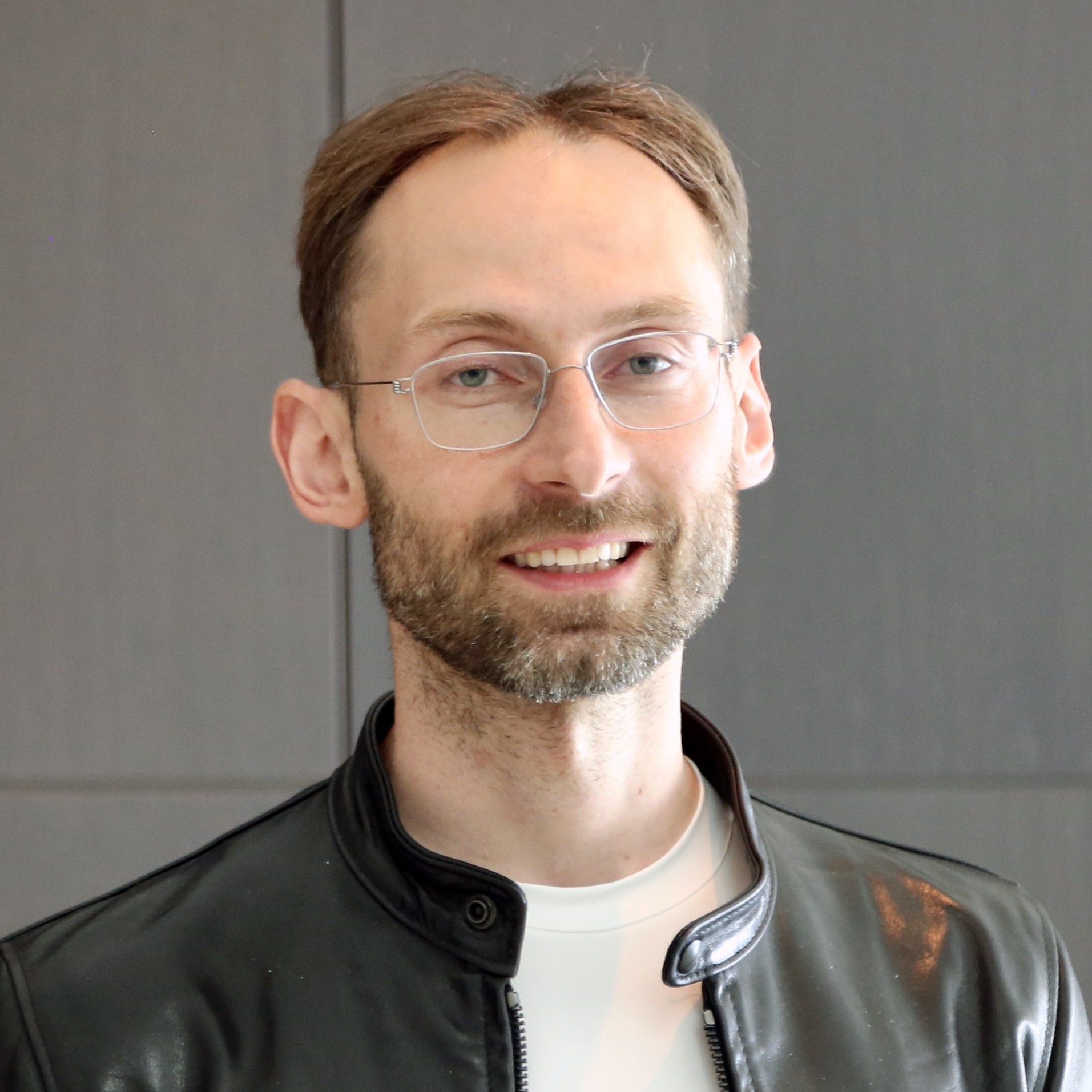
Michael Bronstein
DeepMind Professor of Artificial Intelligence
Department of Computer Science, University of Oxford
Bio
Michael Bronstein is known for coining the term “geometric deep learning” and his research on graph neural networks and 3d shape analysis. He is the DeepMind Professor of AI at the University of Oxford and Head of Graph Learning Research at Twitter. He was previously a professor at Imperial College London and held visiting appointments at Stanford, MIT, and Harvard. Bronstein is the recipient of the Royal Society Wolfson Research Merit Award, Royal Academy of Engineering Silver Medal, five ERC grants, two Google Faculty Research Awards, and two Amazon AWS ML Research Awards. In addition to his academic career, Michael is a serial entrepreneur and founder of multiple startup companies, including Novafora, Invision, and Fabula AI.Geometric Deep Learning: from Euclid to Drug Design
Online series: December 12th (Monday), 16:00 (UTC)
Website Twitter
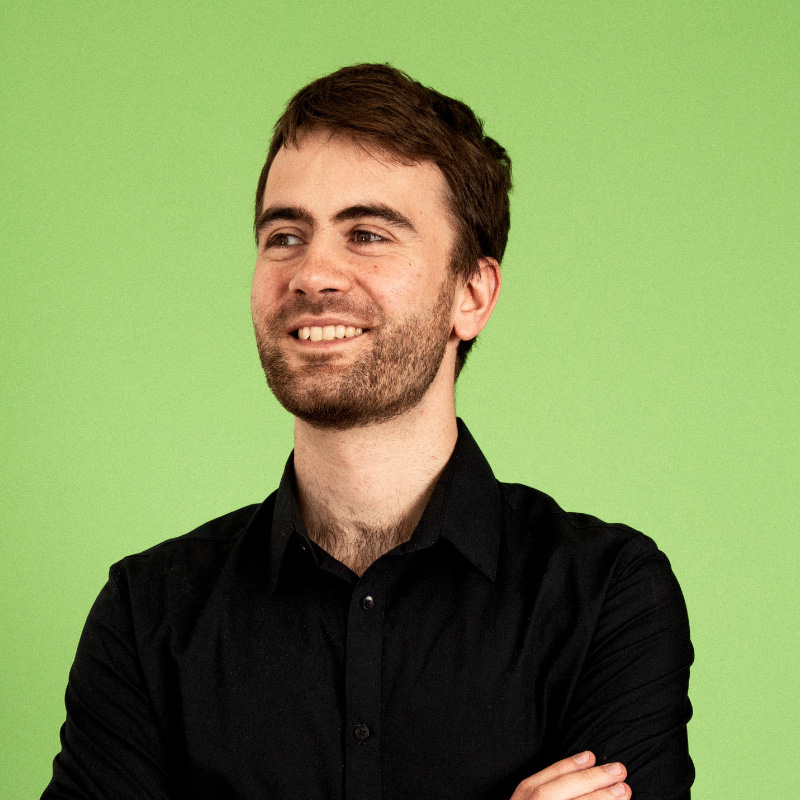
Volodymyr Kuleshov
Assistant Professor
Department of Computer Science, Cornell Tech
Bio
Volodymyr Kuleshov is an Assistant Professor at the Jacobs Technion-Cornell Institute at Cornell Tech and in the Computer Science Department at Cornell University. He obtained his bachelor's in Mathematics and Computer Science from McGill University, and his Ph.D. in Computer Science from Stanford University, where he was the recipient of the Arthur Samuel Best Thesis Award. Kuleshov's research interests are in the field of machine learning and its applications in scientific discovery, health, and sustainability. His work has been featured in Nature Biotechnology, Nature Medicine, Nature Communications and Scientific American, and was awarded an NSERC Post-Graduate Fellowship and a Stanford Graduate Fellowship. He is the co-founder and Chief Technologist at Afresh, a startup focused on automating the food supply chain using AI in order to reduce food waste.Uncertainty-Aware Machine Learning for a Sustainable Food Supply Chain
In the United States, 30–50% of all the food produced is wasted, representing a massive environmental problem—food waste contributes to up to 25% of all greenhouse gas emissions. One of the main drivers of food waste is supply chain inefficiency. This talk describes (1) novel machine learning methods for reasoning under uncertainty and (2) their application to a real-world supply chain control system that significantly improves efficiency and reduces waste. Specifically, I will present improved methods for calibrated uncertainty estimation and planning algorithms that benefit from accurate model uncertainties. This research extends widely used uncertainty quantification methods and demonstrates that most existing planning algorithms can be improved via these methods. I will also describe how these algorithms are deployed in a real-world supply chain control system. This system is currently deployed across hundreds of supermarkets in the US (handling ~10% of US produce volume) and has led to waste reductions of up to 50%.Online series: December 15th (Thursday), 17:30 (UTC)
Website Twitter